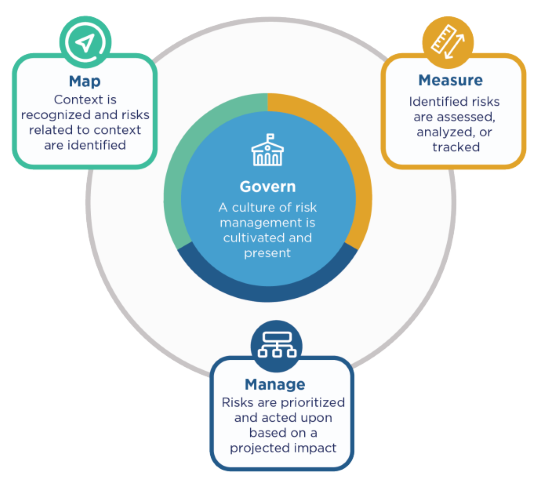
In Part I of our AI ethics series, we highlighted the growing pressure on organizations to adopt comprehensive ethics frameworks and the impact of failing to do so. We emphasized the key motivators for businesses to proactively address potential risks before they become reality.
This article delves into what an AI ethics framework is and why it is vital for mitigating these risks and fostering responsible AI use. We review AI ethics best practices, explore common challenges and pitfalls, and draw insights from the experiences of leading industry players across various sectors. We also discuss key considerations to ensure an effective and actionable AI ethics framework, providing a solid foundation for your journey towards ethical AI implementation.
A comprehensive AI ethics framework offers practitioners a structured guide with established rules and practices, enabling the identification of control points, performance boundaries, responses to deviations, and acceptable risk levels. Such a framework ensures timely ethical decision-making by asking the right questions. Below, we detail the main functions, core components, and key controls necessary for a robust AI ethics framework.
The National Institute of Standards and Technology (NIST) outlines four core functions essential for all AI ethics frameworks. These functions provide the foundation for developing and implementing trustworthy AI:
Figure 1: NIST’s Risk Management Framework (RMF) Core functions for AI
These functions work together continuously throughout the AI lifecycle, enabling effective conversations surrounding the development and implementation of AI technologies (see Figure 1).
Beyond the main functions, an AI ethics framework must include certain core components to be successful. These components ensure the framework is comprehensive and adaptable:
Incorporating these components helps organizations build a resilient and effective AI ethics framework that aligns with their strategic goals and ethical standards.
To ensure the effectiveness and trustworthiness of the AI system, it is important to consider specific key controls when building your AI ethics framework. These controls, integral to the "Measure" function, provide necessary oversight and protection:
Integrating these key controls into the AI ethics framework can ensure that organizations build AI systems which operate as intended and maintain the highest ethical standards.
By understanding and implementing the main functions, core components, and key controls, organizations can create a comprehensive and cohesive AI ethics framework. This framework will safeguard long-term success, foster trust, and ensure the responsible use of AI technologies, ultimately benefiting both the organization and society at large.
Comparing current AI ethics frameworks across private industry, government, and academia reveals varied approaches yet common themes. Predominantly, frameworks emphasize fairness, privacy, governance, and transparency, although depth and structure vary significantly.
Across sectors today, three common categories of AI ethics frameworks exist:
While these three categories are prevalent, the following outlines where organizations are particularly excelling in their AI ethics approaches.
Microsoft's AI ethics framework, building off NIST’s AI Risk Management Framework, stands out for its comprehensive and detailed approach. Their 27-page Responsible AI Standard provides a robust set of resources that detail:
For example, Microsoft has created six goal types, each broken down into further subgoals, which outline the requirements needed to meet each subgoal (see Figure 2). This detailed approach ensures that ethical considerations are systematically measured and addressed throughout the AI lifecycle, promoting responsible innovation and ethical AI deployment.
Figure 2: Microsoft’s Responsible AI Accountability Goal A1
The European Commission also excels in measurement by providing a comprehensive framework that addresses the structure, measurement, and governance of ethical AI. While many government entities outline aspirations for future AI ethical frameworks, the European Commission provides concrete guidance and systematic measurement, ensuring that ethical principles are integrated into AI development and deployment.
The European Commission successfully provides guidelines for ethical and robust AI by making legal obligations mandatory in the development, deployment, and use of AI. They define the foundations of trustworthy AI and principles that must be followed to be considered ethical, turn these principles into seven key requirements needed throughout the AI’s lifecycle, and create an assessment to operationalize trustworthy AI. This legal framework ensures that ethical considerations are not just aspirational but legally binding, providing a robust foundation for trustworthy AI.
Academic institutions are also contributing to legal mandates in AI ethics. Carnegie Mellon University's Responsible AI initiative at the Block Center translates research into policy and social impact, fostering educational and collaborative partnerships. These efforts help shape legal and regulatory standards, ensuring that AI technologies adhere to societal values and enhance human capabilities.
Carnegie Mellon University's Responsible AI Initiative
These efforts by both legal bodies and academic institutions ensure that AI technologies are developed and used responsibly, aligning with societal values and enhancing human capabilities.
MIT excels in fostering a comprehensive and pragmatic approach to governance and regulatory standards in AI ethics. Their AI Policy Brief presents clear principles for ethical AI that prioritize security, privacy, and equitable benefits. MIT advocates for robust oversight mechanisms to ensure responsible AI deployment and emphasizes the need for extending existing legal frameworks to AI. This approach ensures that AI remains safe, fair, and aligned with democratic values.
Key Principles from MIT's AI Policy Brief
MIT underscores the importance of governance structures in maintaining accountability and compliance in AI systems. By integrating ethical principles within a structured but adaptable framework, MIT highlights the crucial role of governance in AI ethics. This approach ensures that AI technologies are effectively monitored and regulated, adapting to technological advancements while safeguarding ethical standards.
These efforts by MIT reinforce the necessity for a regulatory approach that evolves in tandem with technological progress, ensuring that AI development and deployment remain aligned with societal values and democratic principles.
Stanford University's Human-Centered Artificial Intelligence (HAI) initiative stands out for its commitment to steering AI development to enhance human capabilities rather than replace them. Their focus on upholding integrity, balance, and interdisciplinary research ensures that AI technologies are developed with a keen sense of their societal impact. Key aspects of HAI's approach include:
Key Principles of HAI
By emphasizing human-centric development, Stanford’s HAI promotes responsible AI that promises shared prosperity and adherence to civic values. This approach ensures that AI technologies are developed with the primary goal of benefiting humanity and upholding ethical standards.
Current State of AI Ethics Frameworks
Overall, most frameworks are still in a developmental phase, emphasizing general considerations over precise implementation guidelines. Common issues include:
By addressing these inconsistencies and integrating best practices from various entities, organizations can achieve robust and cohesive AI ethics strategies.
Across all sectors, AI ethics frameworks consistently encounter common pitfalls, including missing fundamental components, incorrectly interpreting controls, or lacking sufficient detail on controls. Identifying and addressing these pitfalls is crucial for the effective implementation of AI ethics.
The nature of generative AI makes quantifiable measurement extremely difficult, especially on ethical concerns due to the inherent complexity, subjectivity, and dynamic nature of AI. Traditional machine learning algorithms have standardized methods for measuring accuracy and precision. In contrast, generative AI outputs are ever-changing and non-quantitative, such as text or images, making standard measurement challenging. Ensuring compliance with ethical and bias considerations adds another layer of complexity, often requiring human review.
Mitigation Tactic: Develop systematic measurement guidelines and metrics for decision-making to effectively address these challenges.
Many frameworks lack testing or validation processes, resulting in companies facing issues with inappropriate outputs from AI systems. For example, in February 2024, Google was forced to apologize after users started seeing unexpected results when using its publicly released Gemini AI tool. Image prompts involving specific groups of people resulted in offensive outputs, leading Google to disable the ability to generate images of people entirely. For months afterward, any request to generate an image that involved people would be met with: “We are working to improve Gemini’s ability to generate images of people. We expect this feature to return soon and will notify you in release updates when it does.” This incident highlights the need for more rigorous evaluation processes. Institutions must advocate for and participate in creating comprehensive validation frameworks that include diverse and extensive testing scenarios.
Mitigation Tactic: Implement rigorous evaluation processes to prevent incidents and ensure ethical AI deployment.
Another significant issue in many frameworks is the absence of explicit enforcement or oversight mechanisms. Posting AI ethics guidelines is insufficient without incorporating those guidelines into development processes. An organization’s framework must explain what enforcement mechanisms are in place and how they work. Many governmental AI ethics frameworks are aspirational and lack legal consequences. And while legislative and regulatory processes determine legal enforcement, organizations must plan for proper enforcement mechanisms in anticipation of actual codification. Academic institutions can also help by fostering a culture of accountability and collaboration with industry and government partners to ensure ethical guidelines are actionable.
Mitigation Tactic: Establish clear enforcement mechanisms and integrate them into the development processes.
Issues often arise around three categories of controls: privacy, bias, and legality. Either these controls are not present in current frameworks, or there is a misunderstanding of what they entail and how they need to be addressed.
Mitigation Tactic: Correctly understand and incorporate key controls to address privacy, bias, and legal compliance effectively.
Addressing these common pitfalls is essential for developing robust AI ethics frameworks. By establishing comprehensive measurement standards, implementing rigorous evaluation processes, ensuring effective enforcement mechanisms, and correctly understanding and incorporating key controls, organizations can create ethical AI systems that operate responsibly and effectively.
In this paper, we defined what a framework is and examined its importance in mitigating the risks discussed in Part I. We explored challenges and common pitfalls in establishing an effective AI ethics framework, drawing insights from industry leaders and outlining key considerations for successful implementation.
To ensure ethics are central to AI implementation within your organization, it is essential to use a robust and actionable framework. Establishing an ethical AI framework requires concrete steps:
Part III of this series provides the A&MPLIFY roadmap, a comprehensive guide to help your business implement ethical AI practices. This roadmap will equip you with the tools and strategies needed to integrate ethics seamlessly into your AI initiatives, ensuring responsible and sustainable growth.